Artificial Intelligence (AI) is a trending “buzzword” in the print industry. Taylor Sinclair takes an in-depth look at some of the applications of AI within different phases of the print process.
The world and the industry are changing rapidly, but one thing that can confidently be predicted as being here to stay in the evolving print landscape is AI. Print is moving into a new era, and AI has the potential to be a powerful tool that helps transform automation and boost productivity in print shops.
There is an ever-expanding range of AI-based solutions coming into the print industry. One such company is Neural Vision – a Polish company that was born in the heart of a big flexo converter/printer, equipped with four Comexi printing presses, one W&H Miraflex press, six laminators and a similar number of slitters.
The main problems faced by production director (later co-founder of Neural Vision) Dmitriy Konoiko were quality issues and equipment inefficiency caused largely by human factors. The company had modern inspection systems like BST and AVT for detecting print defects, but still there was potential for operators to make a mistake.
Dmitriy Konoiko called on his brother Alexey, an IT specialist in the AI and Machine Learning (ML) field, who was to become a Neural Vision co-founder as well. Alexey Konoiko offered some solutions, and converters looking to offer efficient accuracy. Delivering advanced production facility analytics, it subsequent AI-based Neural Vision products Axon S and Synapse OEE were created.
The results were apparent within the first month of implementation, and Neural Vision says its solutions have reduced the number of print defects for its parent printer/converter by three times after several years of use.
The company has three AI/ ML based products that it says will eliminate human errors and therefore improve production quality and efficiency management. These products are Axon, an AI system for quality control; Synapse, an AI system for advanced production facility analytics; and Plex, an ML system for data analysis.
Its Axon product has three variations Axon S, Axon P, and Axon L, with the P and L products still under development. Neural Vision describes Axon as an “autonomous system for detecting unprinted areas during the slitting stage in the technological cycle of flexographic production”. Based on a vision system with constantly evolving machine learning, it delivers real-time quality control and prevents the inclusion of unprinted areas in finished flexible packaging.
Synapse is described by Neural Vision as a “gamechanger” for offers autonomous identification of production cycles, based on telemetry received from cameras and sensors installed on production equipment.
Neural Vision says it is moving forward and offering these solutions to the market and has also started co-operating with equipment manufacturers in order to implement its technology into printing and converting machines.
AI-based approval
Moving over to South America, Brazil-based Neuraflow Predictive Media offers an AI-based solution which aims to remove heuristic factors such as personal beliefs, the fear of not being aligned with the team, and decision-making based on history, from the approval process of printed materials.
Co-founders Aislan Baer and Andrê Gazineu describe Neuraflow as a combination of convolutional neural networks, vision transformers (ViT), a proprietary algorithm integrated with generative AI, and a database of eye-tracking analyses. That might sound complicated. However, to sum the company up, Neuraflow’s goal is to eliminate human bias in the evaluation and decision process on whether printed material complies with standards – ultimately leaving the judgment to the machine.
The company’s solutions are to be used as a tool to work in tandem with a print expert’s judgement, not to be used independently, it explains. This is because, as the company puts it, “the real strength of AI lies in the continuous expansion of human capacity, promoting more informed, agile, and effective decisions, while preserving the critical, creative, and interpretive touch that only human beings can provide”.
This was evident to Neuraflow following a study which investigated how ViT architecture could be applied to enhance the evaluation of print samples related to flexography (or any other printing methods).
With the aim of comparing the AI results with human criteria and evaluating AI’s ability to offer additional insights, 30 print samples were analysed, using Neuraflow’s API, which the company claims is the f irst that allows associations between textual descriptions and visual representations. The API is based on the “Vision-Encoder-Decoder Model”, a hybrid architecture that combines a visual encoder and a textual decoder. The combination enables the AI to process high resolution images, identify technical faults, and produce detailed reports on the samples.
The system achieved a 73% agreement rate with the human criteria, demonstrating the robustness of AI in identifying technical parameters such as colour registration, print uniformity, and text legibility.
“The real strength of AI lies in the continuous expansion of human capacity, promoting more informed, agile, and effective decisions.” – Neuraflow Predictive Media
Among the analysed cases, the company says the AI’s consistency in aspects such as colour saturation and sharpness of detail stood out.
Neuraflow says it realised that AI can be a powerful tool to enhance and support human judgment. While appreciating “legendary and effective” methods, such as delta E colour analysis, Canny edge detection and thresholding algorithms (used to highlight visual deviations), and registration techniques (such as the use of local descriptors), the company believes that a transition to its AI solution is beneficial. This is because of f laws which it identifies in the methods above, such as the dependence on precise adjustments, and limitations when identifying complex defects. Neuraflow’s solutions, conversely, allow the operator to work in sync with the AI, it says, to speed up production and highlight impairments in print, consequently improving print quality.
Neuraflow says its ComplyScan module, that reviews texts on packaging and labels and checks for non-compliance or anomalies in legal texts, is being sold directly to converters in Brazil. An AI module for assessing design success when launching or relaunching a product has been primarily commercialised to brand owners, although some converters are also purchasing it and offering it via API to their clients as an additional service. Global pre media companies are also said to be evaluating or testing the technology, with an extra degree of automation needing to be built in due to the high volumes of file processing these companies require.
Transforming packaging
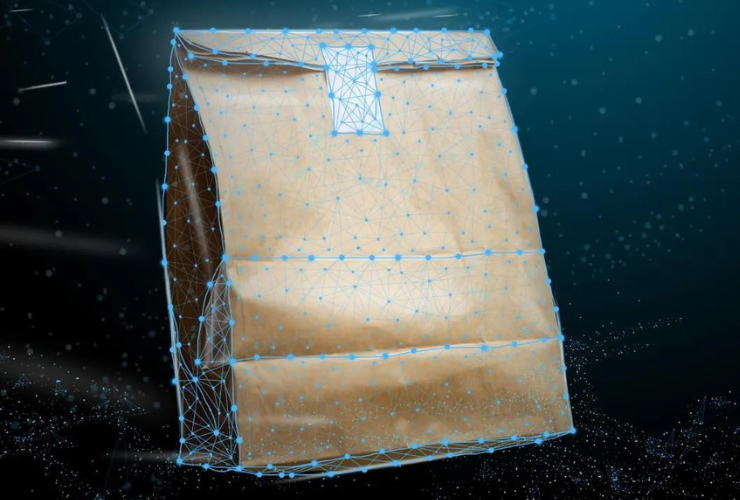
Packaging development incorporates AI, using one.five’s copilot technology
Packaging company one.five has an AI-driven copilot which it says transforms its packaging development, enabling the company to compete on an equal footing with much larger players.
One.five says its AI copilot is designed to work alongside an operator, blending human expertise with advanced machine learning to accelerate packaging innovation while ensuring better customer outcomes.
Claire Hae-Min Gusko, founder of one.five, says its solution addresses the juxtaposing goals of being sustainable whilst remaining affordable. Ms Gusko adds; “There are so many competing factors that a human developer would struggle to manage them all effectively. By programming these factors into a model, we can better identify which aspects to focus on today and where to direct our future efforts. Our goal is to minimise resource inefficiencies as much as possible.”
The company’s AI-driven approach allows it to simulate various conditions – such as those found in form, fill, and seal machines – and material combinations, before carrying out real-world testing. This saves materials and reduces costs, addressing the previously mentioned issue of balancing sustainability and profitability.
By showcasing the data gathered via its AI copilot to customers, the company is able to provide a visual layer of understanding, offering quantitative data to brand customers.
“While the team interacts directly with the AI, customers will experience its benefits through the materials they receive – whether it’s simulations, models, or the final packaging solutions that emerge from the collaboration between the team and the AI copilot,” continues Ms Gusko.
There are challenges ahead. With regulatory changes on the horizon in mainland Europe, particularly in the lead-up to 2028 and 2030, the company believes a “one-fits-all” approach will become outdated, with a shift to packaging solutions tailored to each customer’s unique needs becoming more beneficial. As customers shift towards a tailored approach – considering their supply chain, distribution channels, and even how consumers use the packaging once it reaches their hands – this is where issues may occur for the AI copilot.
“There are so many variables to factor in,” says Ms Gusko. “The challenge lies in how quickly we can gather sufficient data to make reliable predictions or projections about these additional aspects, many of which are relatively new.”